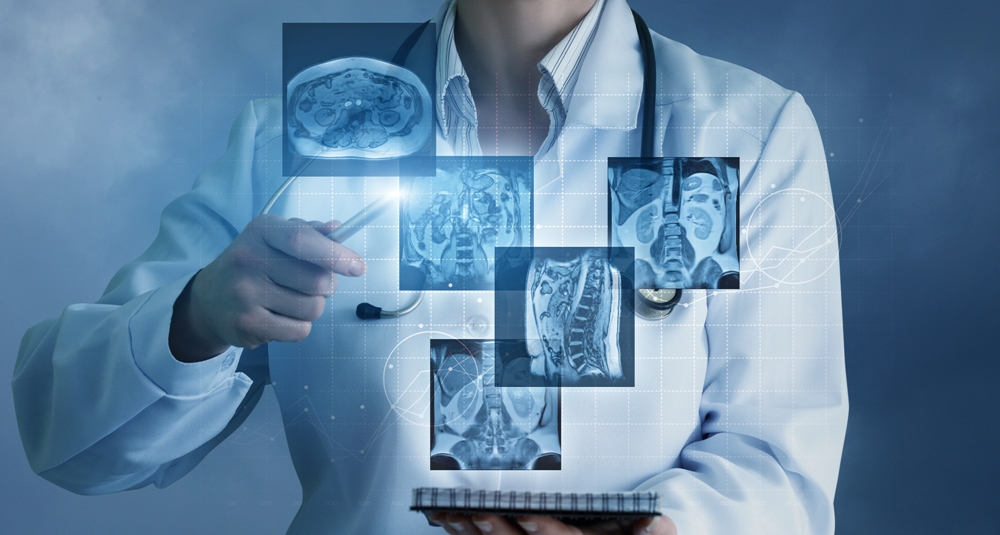
While Hollywood movies and Isaac Asimov predicted a few decades ago that computers would be able to scan out humans from head to toe, real improvements are just starting to surface. There is still no single medical device to detect all health problems, but computer vision can help doctors get closer to that goal.
This is a matter of life and death, since only in the US there are 5.4 million new cases of skin cancer each year, most of which have a 97% survival rate if found early. Since the first examination is only visual and the decision is based on the doctor’s know-how, another informed opinion wouldn’t hurt.
Why Computer Vision?
Computers are excellent assistants when it comes to performing high-volume, structured tasks. The new challenge is to make the machine “understand” what it is looking at by breaking down images into atomic components and analyzing these based on prior indexing. This is done by training neural networks with millions of images in the process called machine learning.
Successful machine learning means that when presented with a new image, similar to those from the training sets, a neural network can identify if there are any health risks, all with excellent accuracy.
Computer vision developers have two main tasks to perform:
- object classification, for segregating new inputs in existing classes for which it was trained, and
- object identification, where the goal is to differentiate between two items belonging to the same group
Applications of Computer Vision in Healthcare
There is no shortage of areas where computer vision could bring groundbreaking innovation to medical imaging: CT, MRI, ultrasound, X-rays, and more are just a few of the use cases.
- X-Rays: The role of X-rays is to identify if there is any abnormalities or damage to a human organ or body part. Computer vision can be trained to classify scan results just like a radiologist would do and pinpoint all potential problems in a single take. This is a healthier approach with radiation exposure limited as much as possible, especially in the case of children and the elderly.
- MRI: While X-rays work best for the bone structure, problems in softer tissues, like joints and the circulatory system, are better highlighted by magnetic resonance imaging (MRI). Training a computer vision system to identify clogged blood vessels and cerebral aneurysms can help save those patients who would be under the radar if the images were analyzed by the naked eye. A group of Japanese researchers proved that this technique could increase aneurysm detection by as much as 13 percent.
- Ultrasound: This is one of the least invasive imaging techniques, used even on pregnant women to follow the fetus development. Using computer vision during pregnancy and for other routine check-ups could help future mothers see if the pregnancy is unfolding naturally or there are any health concerns to take into consideration. Relying on extensive data sets that combine years of medical knowledge, computer vision-equipped ultrasound systems can show more experience than a single physician would. Therefore, prediction accuracy can be significantly enhanced and potential problems identified before they get out of hand.
- CT scans: This method is used to detect tumors, internal bleeding, and other life-threatening conditions. The advantage of using computer vision here is that the entire process can be automated with increased precision, since the machine could identify even those details that are invisible to the human eye. A recent study at the University of Central Florida proved that while trained physicians had only 65% accuracy in detecting lung cancer, the machine was right in 95 percent of the cases. This result becomes even more critical when we are talking about brain damage, strokes, or internal bleeding when every second can make a difference.
Benefits and Challenges of Using Computer Vision in Diagnosis
Usually, time is money; in healthcare, time is life. Using computer vision systems can translate into saving months before learning about life-threatening conditions. In the case of some cancer forms, this could easily be the difference between saving or losing a patient. The benefit of these systems is that they can be trained to spot even slightest abnormalities.
Money is also an important issue here. Every wrong diagnosis translates into additional costs, more tests, more hospital days, and improper treatment, not to mention the psychological impact of potentially bad news that turn out to be false.
Great news about using computer vision is that the same algorithms can be reused for other patients and data from other medical centers can be easily transferred to the system for further algorithm training, thus enhancing the accuracy rates consistently.
The real challenge of training such a system is in finding the right sets of relevant images for training, including of rare cases. To get an excellent accuracy, such training sets should have proper tagging and enough variations to avoid overtraining on simple cases.
Concerns about data privacy and personal security are also on the priority list, but with proper data anonymization techniques, one patient’s data can save the lives of many others.
The futuristic dream of completely automated diagnosis still has countless technical and ethical barriers, but consistent advancements have been made in the last years. AI can be used at various stages of the hospital-patient relationship, from easier admission via chatbots to personalized treatment based on DNA analysis. Medical image analysis is already becoming a field where AI proves to bring groundbreaking results.
Edited by
Ken Briodagh